Machine Learning library Path And Package’s
Machine Learning has emerged as a revolutionary technology for numerous firms today. It’s a tech innovation that ensures swift development of predictive applications. Business owners can leverage the technology and gain crucial insights into organizational data. The analysis and reports will surely help them make informed moves and decisions.
With the help of machine learning, entrepreneurs can perform significant processes such as demand forecasting, fraud detection, and data analytics. Machine Learning comes with reliable insights and powerful algorithms that boost the identification of existing data patterns from existing data sets. These patterns will also help data scientists derive new insights from new information and data sets. Successful data extraction and analytics can be the key to ensuring huge business profits. That’s not all; organizations can identify and solve critical operational issues too.
If you wish to leverage the power of traditional ML and Artificial Intelligence, Pragmatic Machine Learning Toolkit will prove to be the most reliable partner. Data scientists can work on popular ML libraries in Amazon Cloud. Organization owners can scale up their processes and Pentaho Instance size according to their performance needs.
- Pragmatic Deep Learning and Machine Learning Toolkit finds application in the creation of predictive applications. You can use the toolkit for building applications for flagging suspicious transactions, detecting fraudulent orders, forecasting demands, predicting user activities, filtering reviews, analyzing free texts, recommending items, and listening to social media feeds
- Pragmatic offers Pragmatic Deep Learning and Machine Learning Toolkit on AWS marketplace. With some of the major ML libraries including Panda, NLTK, Theano, Tensorflow, Torch, Gensim, Elastics, Spark, and CNTK, customers can reap the benefits of single-click server launch
- By launching an email helpdesk for Pragmatic Deep Learning and Machine Learning Toolkit, Pragmatic helps users solve issues while using and configuring ML libraries. We pride on our associations with efficient data scientists and ML experts, who will understand your problems and offer the perfect solutions to those issues. With in-depth knowledge of Python and ML algorithms, they can work on any predictive analysis issue
- The technology is designed for 24*7 availability. Users won’t come across scheduled maintenance or downtimes. The evaluation, batch prediction, and model training API run on Amazon’s secured, highly reliable, and proven data centers. Even if there’s an ‘availability zone outage’ or ‘server failure,' the toolkit will provide optimum fault tolerance with service-stack replications
- There are no charges involved in the process
- It’s T2 Medium with a storage capacity of 50GB
Here are the essential features of this innovative toolkit:
- OS: Ubuntu 16.04 LTS
- Installed Libraries: Pandas, NLTK, Scikit-learn, Theano, TensorFlow, CAFFE, TORCH, Spark, Gensim, Elastics, CNTK
- Python: - Python2/3
- You want to draw conclusions from your data that help you solve a particular problem. The typical skills of a data scientists are
ML algorithms work on data models. It does not formulate manual rules but learns the entire data model. As a combination of computer science, mathematics, statistics, computational, and quantitative analyses, data science provides the impetus for better and improved decision making. ML or Machine Learning principles are integral parts of ‘data science’ projects. It finds application in the discovery of clustering algorithms and exploratory analysis. Data engineering happens to be a significant part of data science too, and it involves the collection, cleaning, and wrangling of crucial data sets. Data scientists will draw inferences from specific data sets that help them solve particular issues. The standard and common skills of data scientists include - Computer Science: Programming, hardware expertise
- Math: Calculus, Linear algebra, Statistics
- Communication: Presentation and Visualization
- Domain knowledge
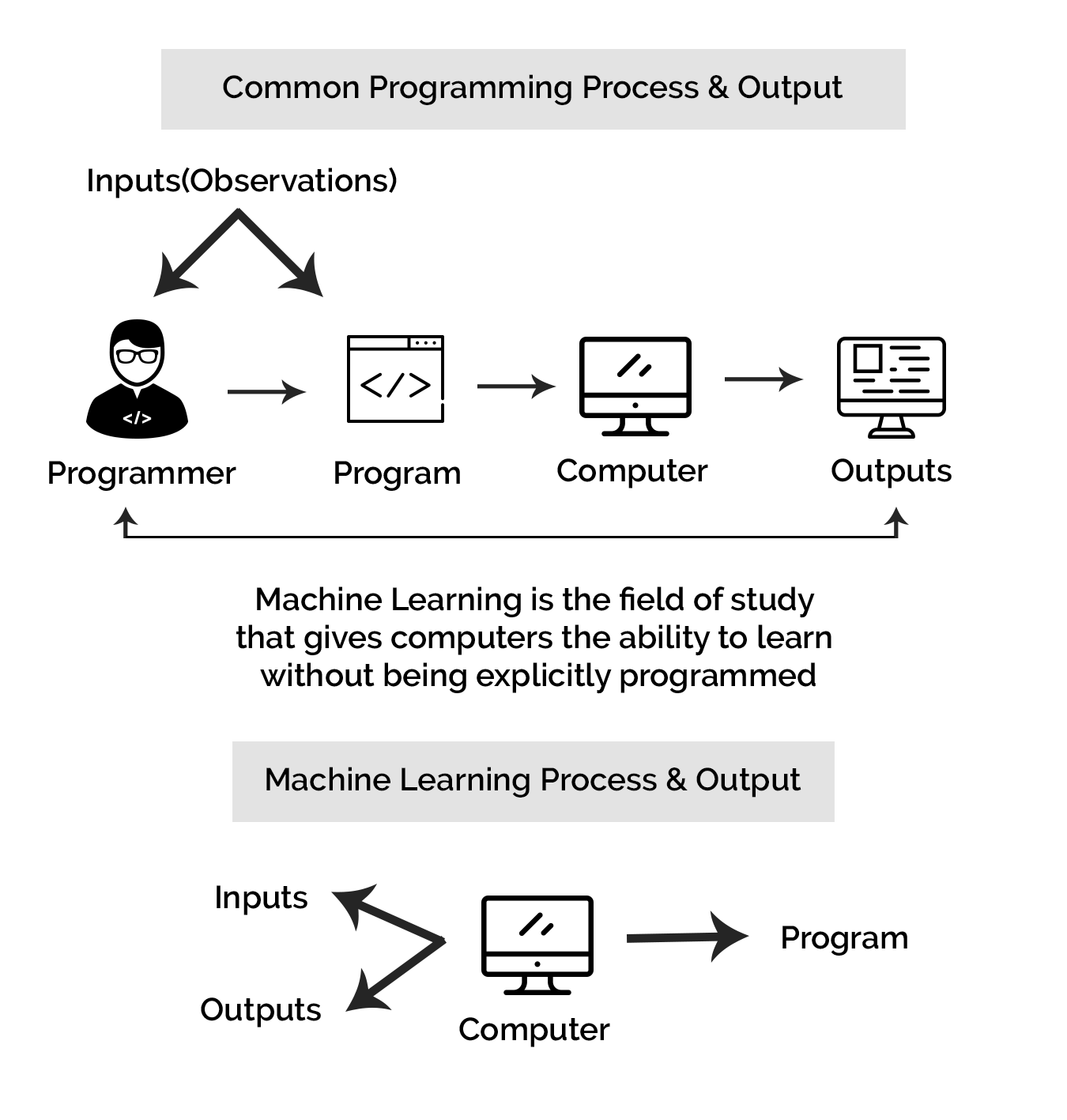
Johnny-Five
Johnny_five library pathLibrary name:- node_modules
ubuntu@ip-x-x-x-x:~$ cd /opt/
Now open your text editor and create a new file called "johnny_five_test.js", in that file type or paste the following:
var five = require("johnny-five"),
board = new five.Board();
board.on("ready", function() {
// Create an Led on pin 13
var led = new five.Led(13);
// Strobe the pin on/off, defaults to 100ms phases
led.strobe();
});
Make sure the board is plugged into your host machine (desktop, laptop, raspberry pi, etc). Now, in your terminal, type or paste the following:
Should run below command through root privilege or sudo users
ubuntu@ip-x-x-x-x:~$ sudo node johnny_five_test.js

After run the above js script. Success should look like this
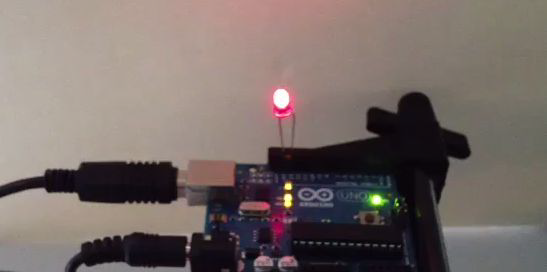
Pandas
/usr/local/lib/python2.7/dist-packages/pandas
#sudo pip install xlrd
#sudo pip install xlwt
#sudo pip install openpyxl
#sudo pip install XlsxWriter
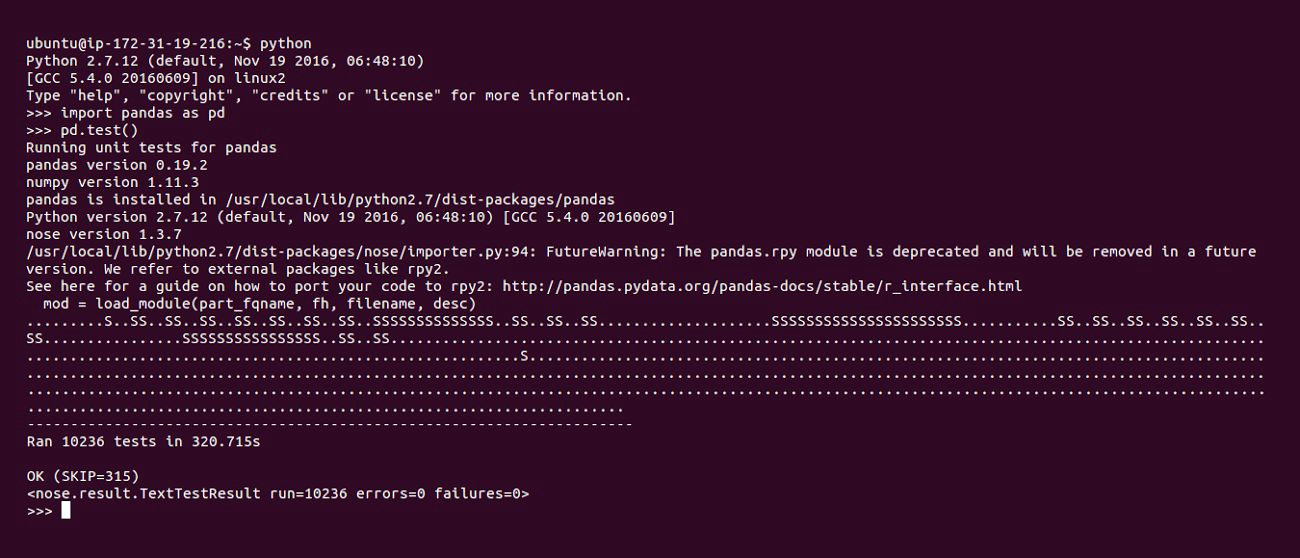
Scikit-learn
/usr/local/lib/python2.7/dist-packages/scikit_learn-0.18.1.dist-info
/usr/local/lib/python2.7/dist-packages/scikit_image-0.12.3.dist-info
Testing requires having the nose library. After installation, the package can be tested by executing from outside the source directory:
nosetests -v sklearn

NLTK
/usr/lib/python2.7/dist-packages/nltk
Theano
/usr/local/lib/python2.7/dist-packages/theano
python `python -c "import os, theano; print(os.path.dirname(theano.__file__))"`/misc/check_blas.py
run the Theano/BLAS speed test:
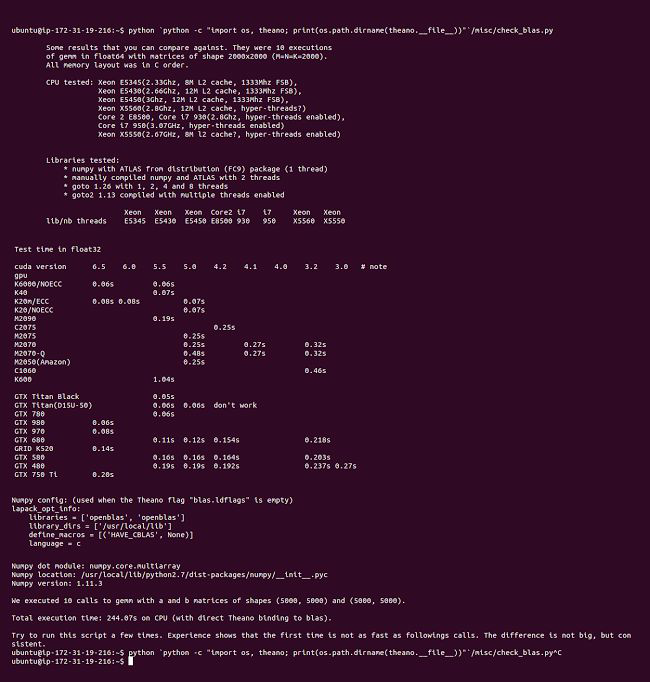
CAFFE
/opt/caffe
TensorFlow
/usr/local/lib/python2.7/dist-packages/tensorflow
TORCH
Generating OpenBLASConfigVersion.cmake in/opt/OpenBLAS/lib/cmake/openblas
Install OK!
/opt/torch
/home/ubuntu/.bashrc

Spark
/usr/lib/spark/python/pyspark
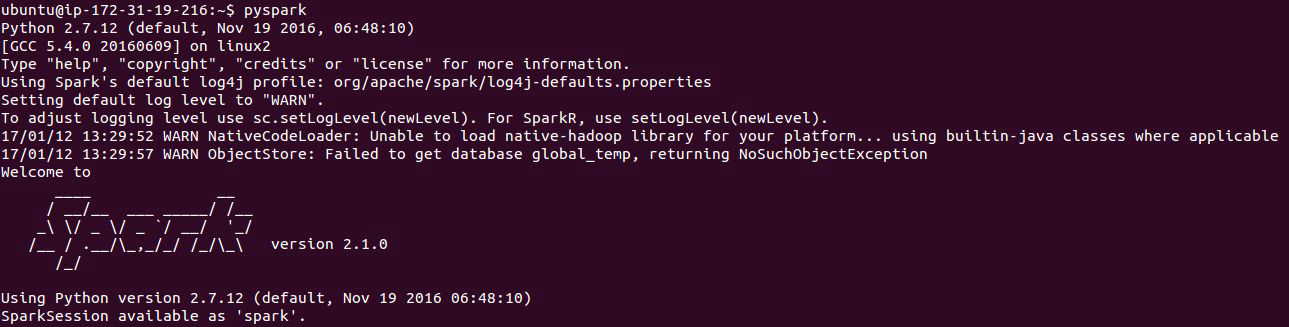
Gensim
/usr/local/lib/python2.7/dist-packages/gensim
Elastics
/usr/share/elasticsearch/lib

CNTK
/usr/local/CNTKCustomMKL
To activate the CNTK environment, run
source
"/home/ubuntu/cntk/activate-cntk"
Please checkout tutorials and examples here:
/home/ubuntu/cntk/Tutorials
/home/ubuntu/cntk/Examples

Johnny-Five
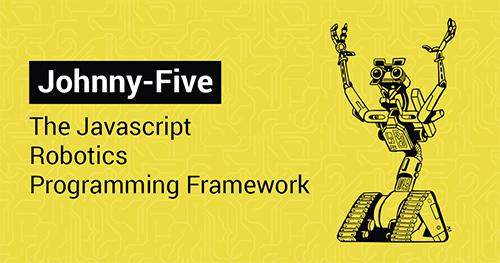
Pandas
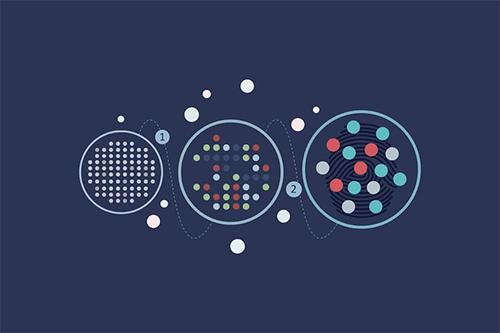
CNTK
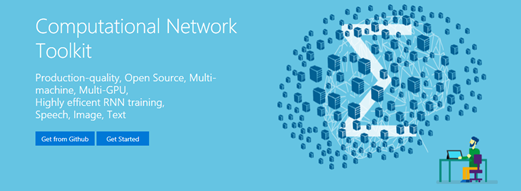
Although there’s a tremendous competition between CNTK and Google’s TensorFlow tool, skilled coders and programmers prefer the former framework to the latter. As far as program documentation is concerned, both these tools need improvement. Since it runs on Windows, CNTK offers better functionality and operability than its competitor.
Scikit-learn
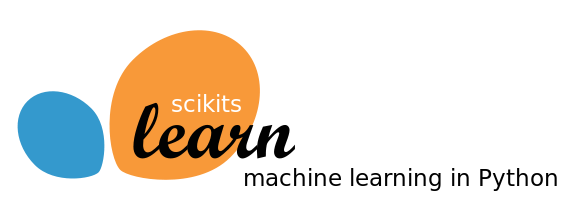
NLTK
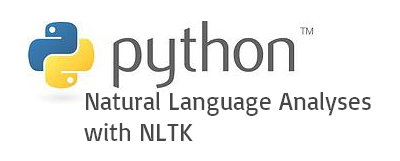
Originally developed at the Pennsylvania University, NLTK finds application in diverse modules and courses in 32 universities worldwide. Some of the highlights of this particular library include:
- Lexical analysis
- Text-and-word tokenization
- Collocations and n-gram
- Parts-of-speech tagging
- Text-chunker
- Tree Model
- Named-entity recognition
TensorFlow
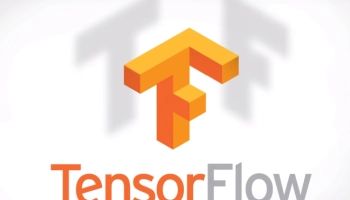
Theano

CAFFE
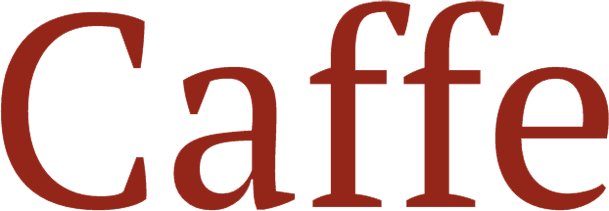
TORCH
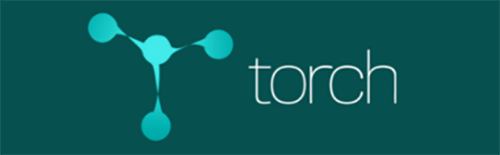
- Computer Vision
- Community-created packages
- Signal processing
- Image and video processing
- Parallel processing
- Networking
- Builds with the Lua community
Spark
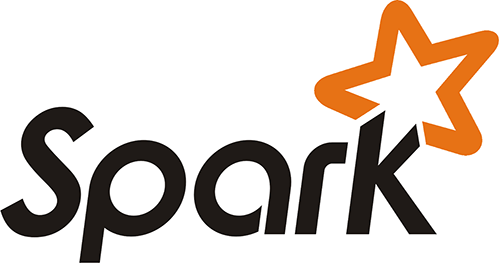
Gensim
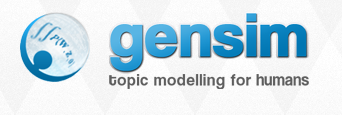
Elastics
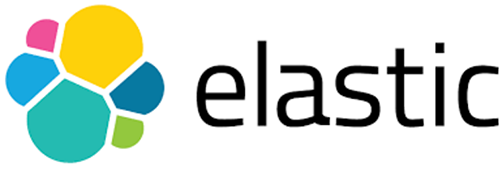